How Machine Learning Helps Prevent Ad Fraud
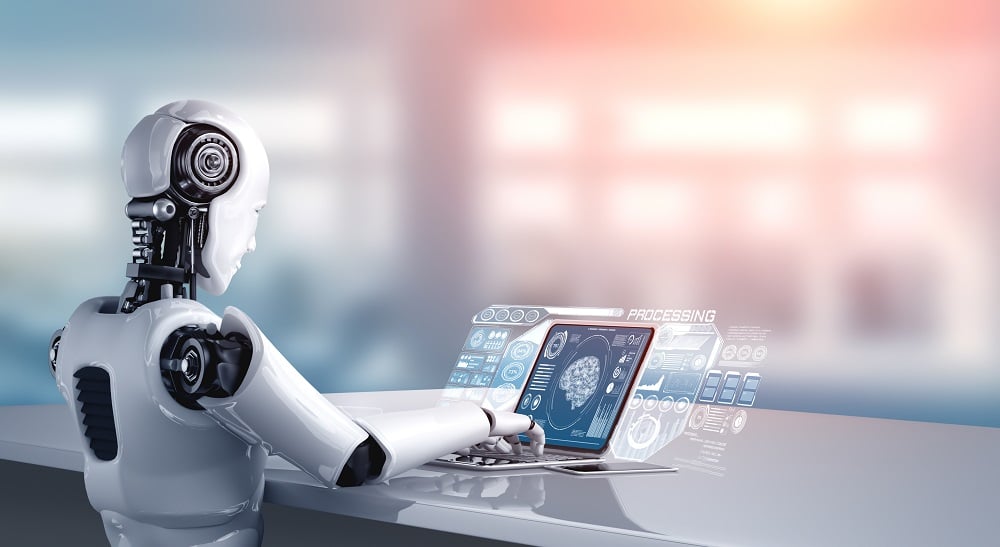

Wherever there’s money to be made, you’ll find fraudsters, too. If you work in the digital advertising industry, you’ll likely have been left frustrated by what seems like a never-ending stream of fraudulent attacks on your efforts.
You’ll also have likely realized that no matter what you do, or how many solutions you put in place, the scammers are always one step ahead of you. Ultimately, you’ve learned that your homemade blacklists and even the most sophisticated of password protectors can’t protect your organization against the prevalence of ad fraud.
Machine learning has been a popular buzzword spanning many of the 2010s and has recently gained traction as a major contender in the fight against ad fraud. Juniper Research suggests that by 2022, machine learning could save advertisers over $10 billion in ad spend that would have been lost to ad fraud. Additionally, leading research firm Gartner agrees that machine learning is a critical component in fighting online fraud.
Ad fraud is dynamic and constantly evolving. Here we take a look at how machine learning is leading the fight against ad fraud.
Machine learning is proactive against highly present and dynamic ad fraud
Machine learning extracts patterns and identifies relationships within datasets. From this, algorithms are applied to datasets to detect fraud. When these algorithms are applied to new datasets, what they’ve learned from known datasets is applied to new ones. Simply, as new and different data is collected, machine learning automatically recognizes new trends amongst large volumes of data.
Humans can’t replicate the speed of machine learning-based fraud detection and prevention
Why is this helpful? Large amounts of fraud can be disguised amongst large volumes of digital traffic: Machine learning quickly identifies reproduced patterns that are hidden in volumes of data at a rate that’s impossible for humans to replicate.
Let’s dive a little deeper into how machine learning combats dynamic ad fraud.
Provides better contextual analysis
Rules-based anti-fraud solutions are generally static and revolve around predefined knowledge that intend to identify generalized fraud patterns. However, machine learning can take each instance of traffic and analyze its parameters.
For example, machine learning can examine multiple variables -- looking past two-dimensional indicators. This allows for the detection of more instances of fraud and emerging fraud patterns. Rules-based programs are unable to identify changes in traffic behavior in the flexible way machine learning can. However, using a combination of rules-based programs and machine learning ensures that organizations can both accurately detect and prevent digital fraud.
Detects fraud faster
Once developed, machine learning applications can run almost autonomously, unlike systems operated by humans, which tend to be time-intensive. Novel fraud predictions are obtained faster, which means it can be addressed in close to real-time. Whereas rules-based systems require steps for human verification, machine learning can quickly identify subtle pattern modifications.
Essentially, this means that users aren’t waiting until a new method of fraud has become widespread before it’s detected, which in turn means these methods can be mitigated before they drain ad budgets.
👉 Read more: The Essential Guide to Ad Fraud Detection for Marketers & Advertisers
Enhances the sensitivity of fraud detection
Machine learning algorithms can process huge amounts of data points concurrently, and self-train to detect different patterns without much human intervention, increasing the sensitivity of the detection. Moreover, machine learning is less biased with regards to human analysis.
Machine learning accelerates the detection and prevention of ad fraud
Fraud is forever changing, and at a faster rate than ever to avoid detection systems. But machine learning has the benefit of constantly improving to detect evolving fraud at the same rate as it’s created.
Since human behavior is constantly changing -- from geography to demographic variations -- any fraud prevention system needs to be able to adjust and react to these changes to avoid blocking traffic that’s legitimate. However, there are always “triggers” within data that are so subtle, and almost invisible to the human eye, that machine learning can detect.
Machine learning algorithms can also be customized to each organization’s circumstances, which means that its pinpointing of bad traffic and ad fraud is even more accurate.
Prevent and detect ad fraud with a machine learning solution
As digital ad spend continues to rise, so do opportunities for ad fraud. But detecting scammers has become a game of cat and mouse, and without sophisticated solutions, you’ll always be lagging behind them.
Ad fraud is constantly evolving. Preventing sophisticated fraudsters and their methods requires a proactive solution, the likes of which only a combination of both rules-based programs and machine learning can truly address.
If you’d like more details about how Opticks’ anti-fraud solution can apply its advanced research, novel state-of-the-art machine-learning algorithms, and expert rules to help protect your organization, click here to request a demo and find out how we can work together to prevent the damage caused by ad fraud.